By Jan Hoffmeister, Managing Partner at Drooms
Q, the first cinema robot appeared in The Master Mystery in 1919. Ever since then we have seen fictional automatons of various shapes and sizes wreak havoc on the human race.
It’s perhaps not surprising that deep suspicion of the role of robots in business and their impact on the way we work has developed over time. But the truth is that robotics and artificial intelligence (AI) are set to introduce huge benefits to the finance sector in particular.
AI research was founded in a workshop at Dartmouth College in 1956 and those who attended became the leaders of AI research for decades. Their ambitious predictions for a machine that would be as intelligent as a human being attracted generous funding, which led to the development of a robot by the AI centre of Stanford Research Institute called ‘Shakey’ (1966-1972) controlled by a large computer.
By the early 1970s it was clear that AI was in trouble, largely because of hardware limitations. But the doubling of processing power every year under Moore’s Law meant that by 1996 IBM was able to build Deep Blue, a chess-playing computer that famously beat world champion Garry Kasparov.
Fast forward to 2014, and Google invested US$400 million in artificial intelligence start-up DeepMind. While the media discussion about AI has gravitated towards the idea that machines will replace humans and leave them with nothing to do, the fact is that AI is not about to automate most financial professions. Rather, it aims to complement human intelligence.
Recently, Google founded the People + AI Research Initiative (PAIR) to better explore these interactions with the support of researchers from MIT. The financial industry has also understood the potential hidden in the application of AI. Of the 5,000 FinTech start-ups identified by a 2016 report by Ernst & Young, a large number are set to bring intelligence to the banking world.
Fields where AI is particularly relevant include client servicing, trading, post-trade operations such as reconciliations, transaction reporting, tax operation and enterprise risk management.
A study released in 2017 by PwC points to a similar trend: ‘AI will gradually replace humans in some functions like personal assistants, digital labour, and machine learning. But challenges will persist because of bias, privacy, trust, lack of trained staff, and regulatory concerns. Augmented intelligence, in which machines assist humans, could be the near term answer.’ Future decision-making processes in banking, for instance, will be 34% informed by machine algorithms and 66% by human judgment.
A sub-field of AI is a particularly strong example of applications of augmented intelligence – Natural Language Processing. NLP is the development of systems capable of reading and understanding the languages that humans speak.
At the heart of this technology is the ability to interpret a large amount of so-called ‘unstructured data’ (i.e. data that cannot be read by machines yet, such as PDF files, images and audio material). The FinTech sector is leveraging this technology because it significantly improves customer interactions, and not only by implementing chatbots.
The finance industry is a knowledge-intensive sector and key tasks require the reading and understanding of large amounts of information that are only partly structured. NLP is making processes much easier, faster and more precise with less effort than ever from humans.
Start-ups such as MonkeyLearn are already leveraging NLP to automate business intelligence information, whereas leading Japanese asset manager Nomura Asset Management (NAM) are investing to understand whether this technology can improve the decision-making processes of portfolio management’s investors. Even providers of legal services are turning towards NLP, automated document analytics and law case reporting within due diligence processes.
Putting users at the heart of the software was our goal in developing the new virtual data room, Drooms NXG. Aware of the fact that the biggest issue for data room users was to keep up with time-consuming and demanding document analysis, the implementation of the most effective technology was required. As NLP enables the reading and understanding of language, we saw a huge opportunity to apply this new technology to the content of data room documentation.
Today, the data room of Drooms NXG can present information to users thanks to smart pre-set categories, suggested red flags, lists of documents by relevance and recommendations for smarter risk management. When users search their VDR for terms in order to find potential red flags chosen from the categories listed by Drooms NXG Findings Manager, the system returns a ‘Suggested Findings’ list which can be categorised as very low, medium or high level risk or opportunity. Professionals can add their own categories or edit existing keywords to make their searches as focused as possible. Individual calls to action can be assigned to each one; adding an individual note to highlight specific insight, incorporating a link to a document, page or even a paragraph or single word; or using a colour coding system by selecting one of the built-in colour tags. Similarly, we wanted to address language barriers between users. Now, documents can be instantly translated within the data room, thereby facilitating cross-border deals.
These solutions were not developed with the goal of replacing manpower with automated data analysis. On the contrary, our goal is to empower deal experts by developing intuitive tools that don’t feel like a burden, but make their decision-making more accurate and their business lives more successful.
According to McKinsey’s 2017 survey, early AI adopters that combine strong digital capability with proactive strategies have higher profit margins and expect the performance gap with other firms to widen in the next three years. Financial firms should no longer be concerned that the robots are coming, only that they harness the power of robotics and AI to improve the service that they provide to customers.
Website: https://drooms.com
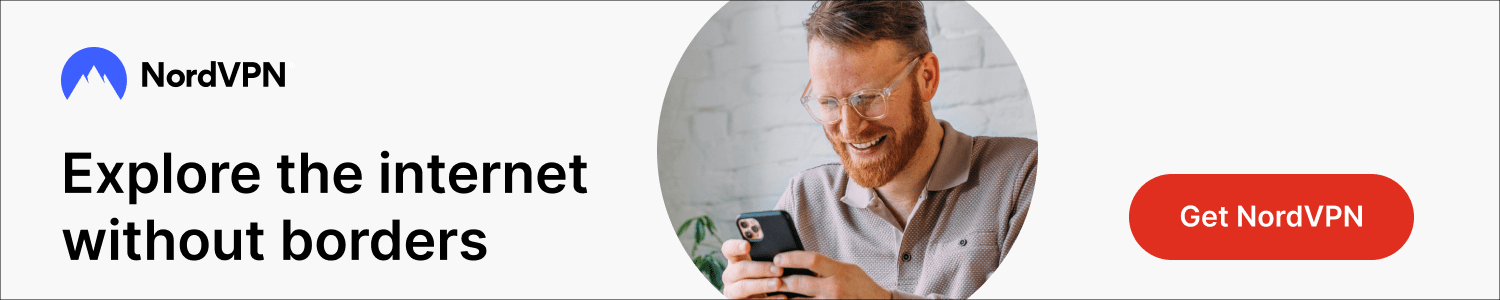