Whether or not the shift is permanent, the convergence of Brexit and the COVID-19 calls for updated machine learning and prediction models in the UK financial sector.
Richard Harmon, Managing Director of Financial Services at Cloudera, discusses the importance of relevant machine learning models in today's age, and how the financial sector can prepare for future changes.
The past six months have been turbulent. Business disruptions and closures are happening at an unprecedented scale and impacting the economy in a profound way. In the financial services sector, S&P Global estimates that this year could quadruple UK bank credit losses. The economic uncertainty in the UK is heightened by Brexit, which will see the UK leave the European Union in 2021. In isolation, Brexit would be a monumentally disruptive event, but when this is conjoined with the COVID-19 crisis, we have a classic double shock wave. The duration of this pandemic is yet to be known, as is the likely future status of society and the global economy. What the ‘new normal’ will be once the pandemic has been controlled is a key topic of discussion and analysis.
It’s not easy to predict the unpredictable
In these circumstances, concerns arise about the accuracy of machine learning (ML) models, with questions flying around regarding the speed at which the UK and EU will recover relative to the rest of the world, and what financial institutions should do to address this. ML models have become essential tools for financial institutions, as the technology has the potential to improve financial outcomes for both businesses and consumers based on data. However, the majority of ML models in production today have been estimated using large volumes and deep histories of granular data. It will take some time for existing models to be re-estimated to adjust to the new reality we are finding ourselves in.
The most recent example of such complications and abnormalities, at a global scale, was the impact on risk and forecasting models during the 2008 financial crisis. Re-adjusting these models is by no means a simple task and there are a number of questions to be taken into consideration when trying to navigate this uncertainty.
ML models have become essential tools for financial institutions, as the technology has the potential to improve financial outcomes for both businesses and consumers based on data.
Firstly, it will need to be determined whether the current situation is a ‘structural change’ or a once in a hundred years ‘tail risk’ event. If the COVID-19 pandemic is considered a one-off tail risk event, then when the world recovers, the global economy, the markets, and businesses will operate in a similar environment to the pre-COVID-19 crisis. The ML challenge, in this case, is to avoid models from becoming biased due to the once-in-a-lifetime COVID-19 event. On the other hand, a ‘structural change’ represents the situation where the pandemic abates, and the world settles into a ‘new normal’ environment that is fundamentally different from the pre-COVID-19 world. This requires institutions to develop entirely new ML models that require sufficient data to capture this new and evolving environment.
There isn’t one right answer that fits every business, but there are a few steps financial services institutions can take to help them navigate this scenario.
How to navigate uncertainty with accurate machine learning
- Modify existing models: This will be the starting point for all data science teams and can involve using the latest data elements to modify current models while also creating scenario-based projections adjusted for various levels of model bias.
- Stress testing: A practice that helps businesses gain a clearer understanding of their hidden vulnerabilities. Finance leaders should work alongside Chief Risk Officers to set up multiple dynamic stress testing scenarios.
- Industrialisation of ML: This is the ideal time to invest in a platform that supports the entire ML life cycle to build, validate, productionise, manage and monitor all their models across the entire enterprise. Data about customers is coming from different places and is stored in different places, whether that’s the public cloud or on-premise. For ML models to be effective, they need to be taking all these streams of data into account, with the ability to understand what the different data is saying quickly. This can only be done effectively in a unified enterprise data cloud platform.
- Prescriptive analytics: This approach is complementary to ML and uses simulations for more accurate decision-making for different scenarios, brought on by shocks or market changes. One common approach is Agent-Based Modeling (ABM), a bottom-up simulation for modelling of complex and adaptive systems. ABMs help business project thousands of future scenarios without having to depend upon the limitations of historical data.
[ymal]
When facing a crisis of unprecedented size such as this one, it’s time to look inwards and review the technology investments in place and whether crucial tools such as ML models are being deployed in the best way possible. Financial institutions should face this issue not as responding to a one-off crisis, but as a chance to implement a longer-term strategy that enables a set of expanded capabilities to help prepare them for the next crisis. Businesses that put in time and effort to re-evaluate their machine learning models now will be setting themselves up for success.
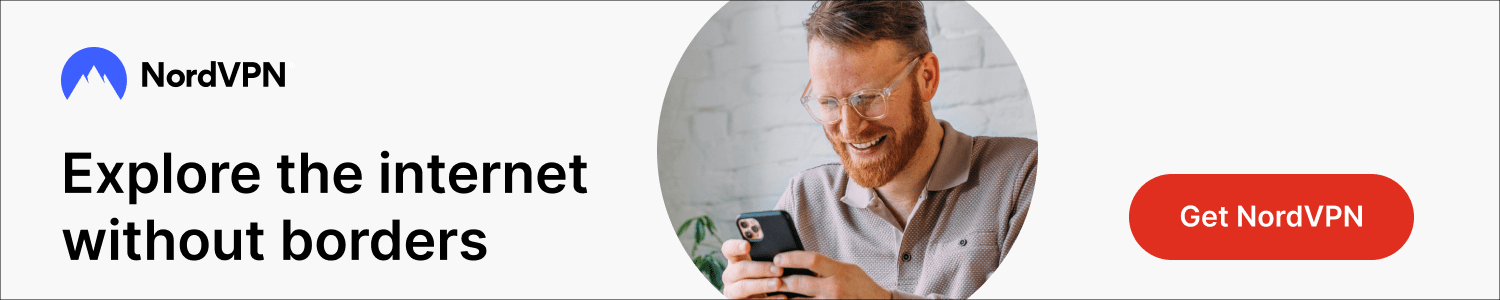